
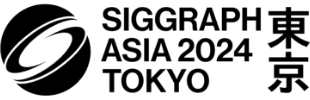
Local Gaussian Density Mixtures
for Unstructured Lumigraph Rendering
Xiuchao Wu1 Jiamin Xu2 Chi Wang1 Yifan Peng3 Qixing Huang4 James Tompkin5 Weiwei Xu1
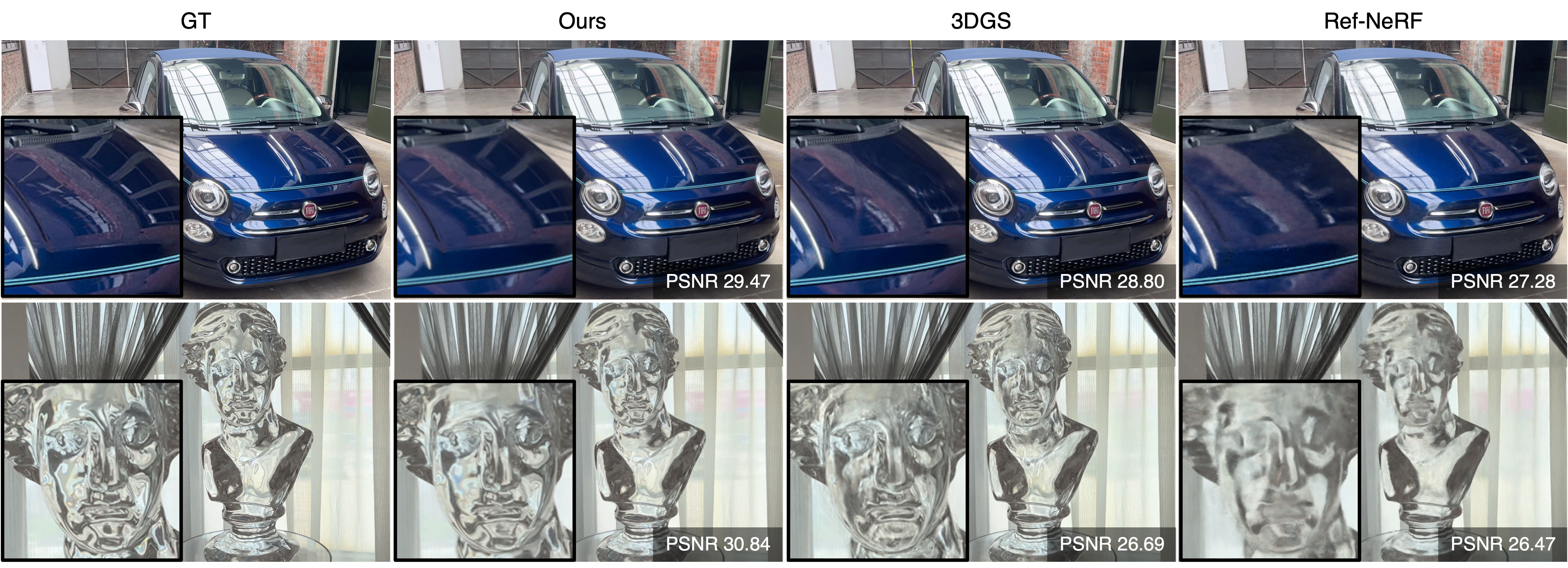
Abstract
To improve novel view synthesis of curved-surface reflections and refractions, we revisit local geometry-guided ray interpolation techniques with modern differentiable rendering and optimization. In contrast to depth or mesh geometries, our approach uses a local or per-view density represented as Gaussian mixtures along each ray. To synthesize novel views, we warp and fuse local volumes, then alpha-composite using input photograph ray colors from a small set of neighboring images. For fusion, we use a neural blending weight from a shallow MLP. We optimize the local Gaussian density mixtures using both a reconstruction loss and a consistency loss. The consistency loss, based on per-ray KL-divergence, encourages more accurate geometry reconstruction. In scenes with complex reflections captured in our LGDM dataset, the experimental results show that our method outperforms state-of-the-art novel view synthesis methods by 12.2% - 37.1% in PSNR, due to its ability to maintain sharper view-dependent appearances.
Rendering Results (LGDM dataset)
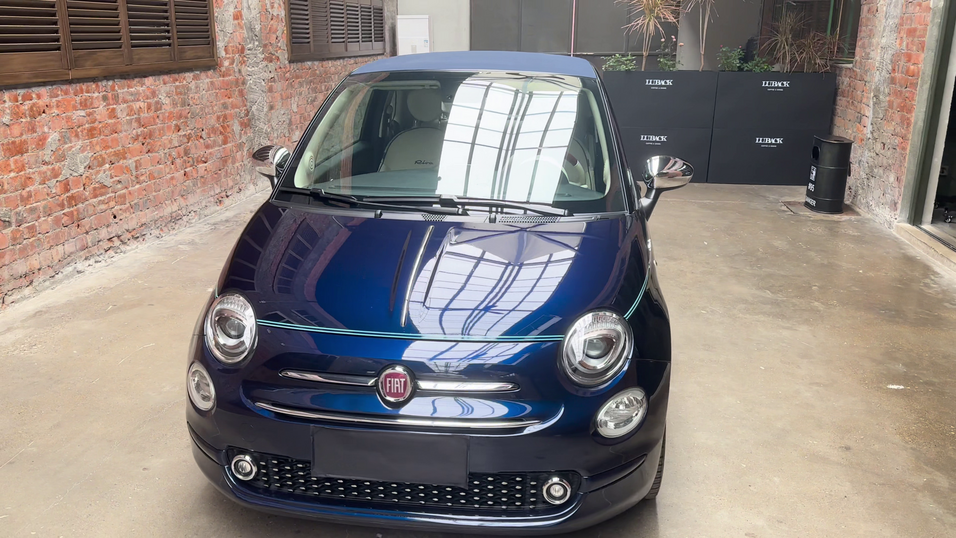
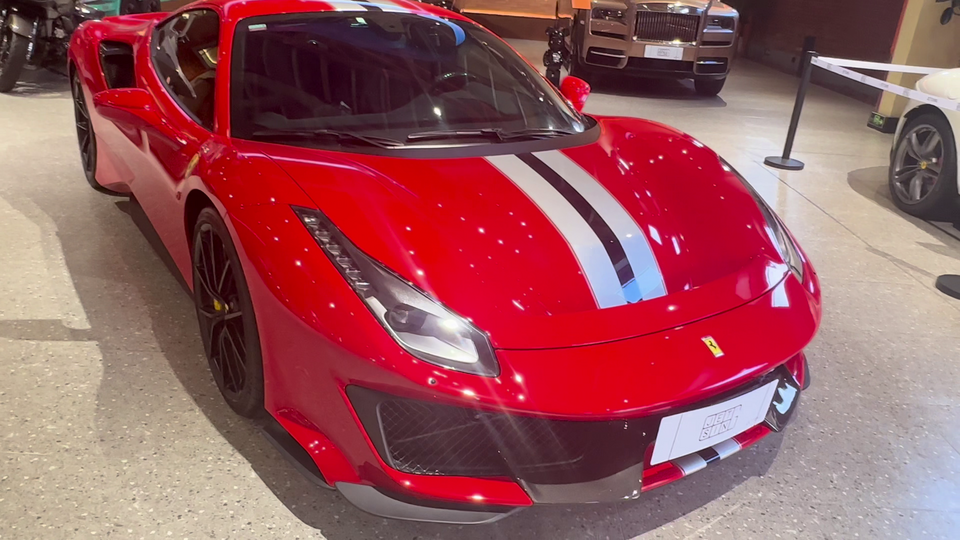
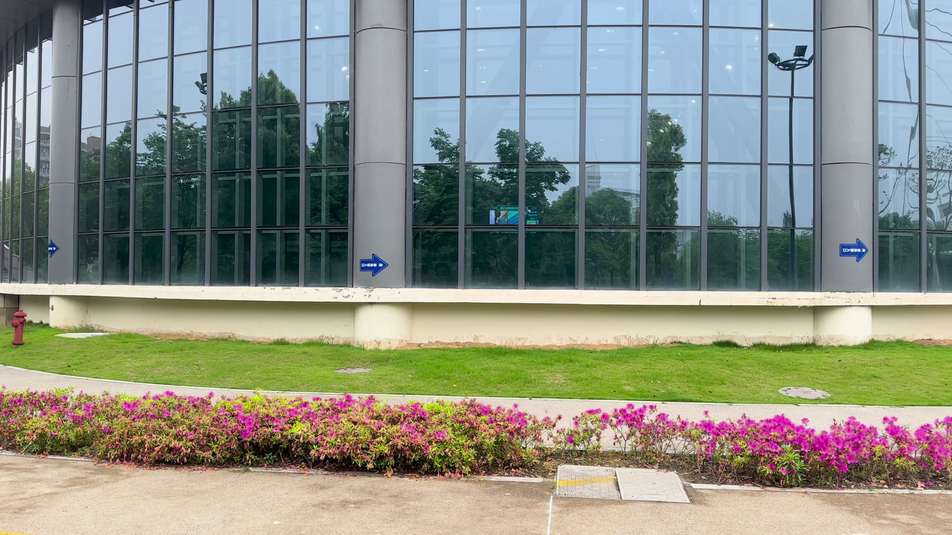
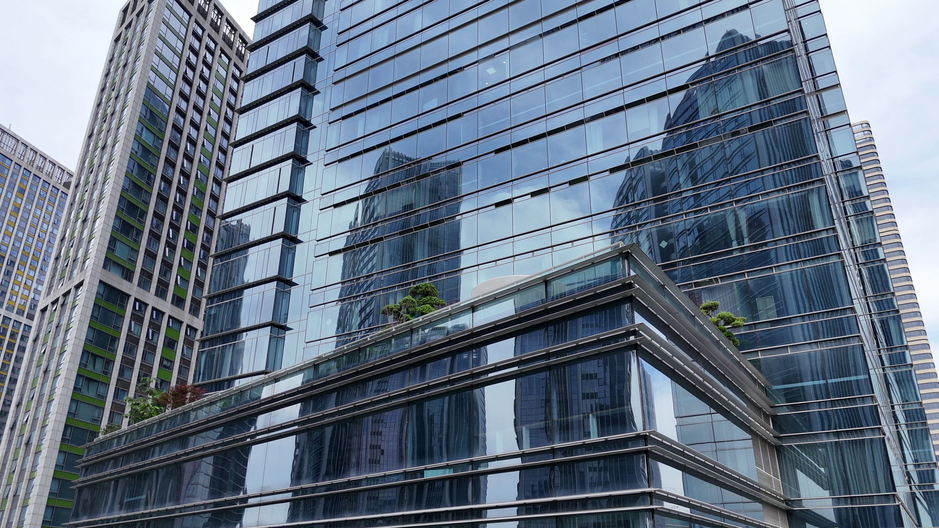
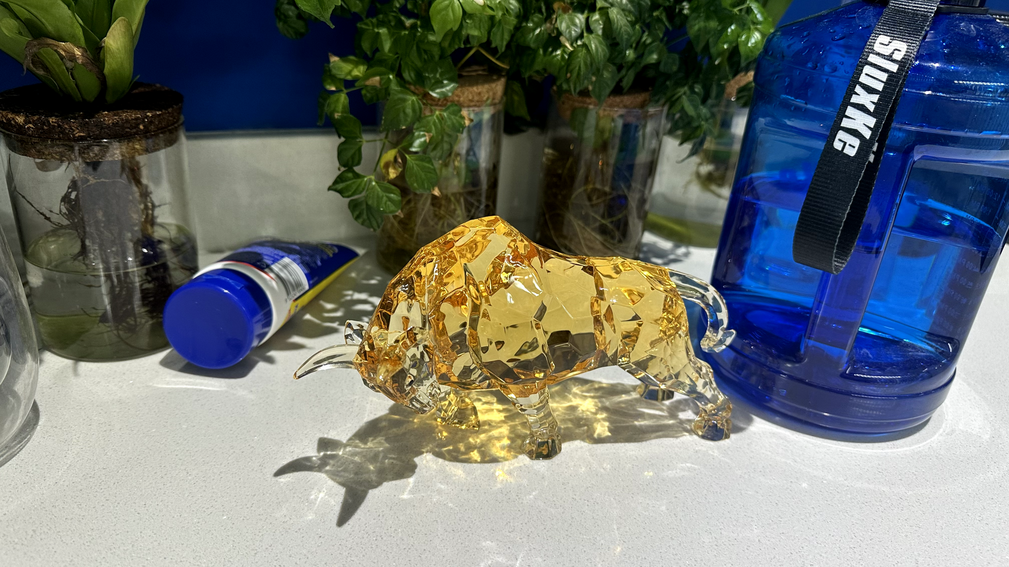
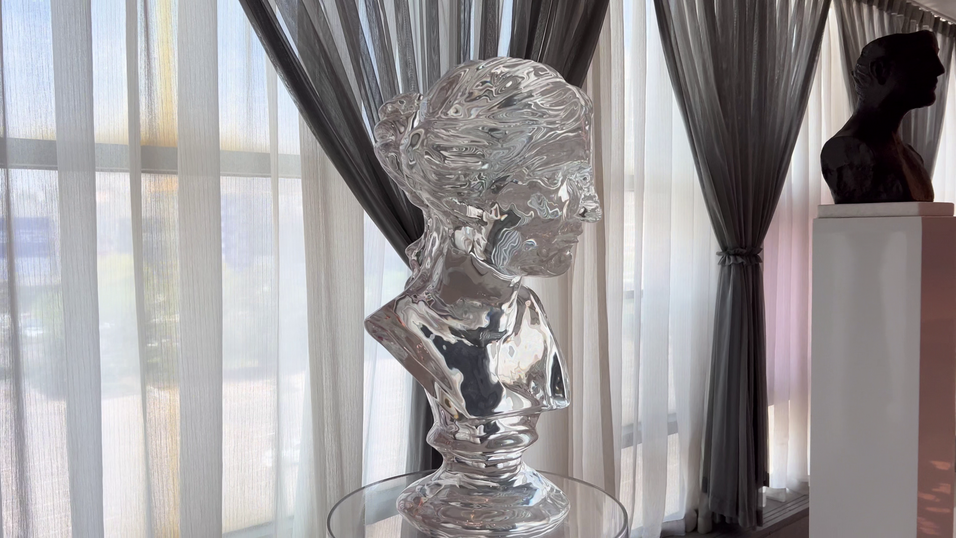
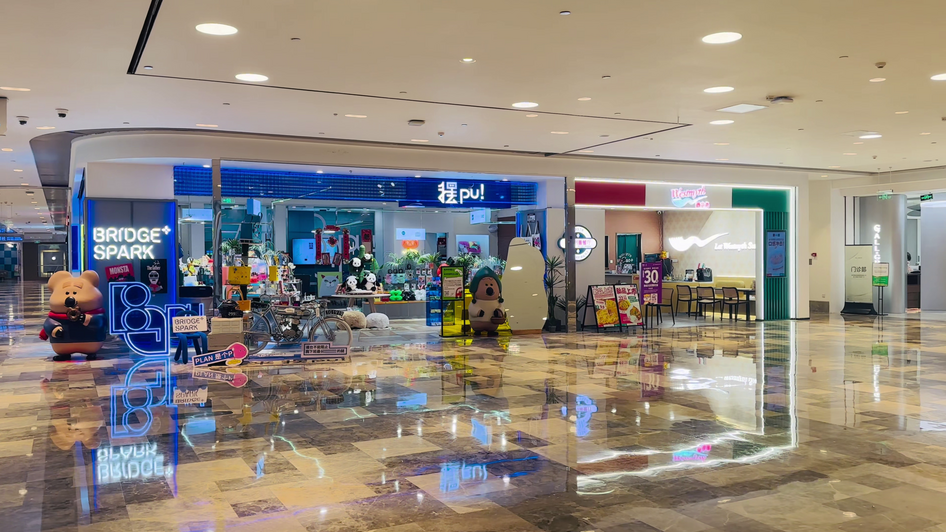
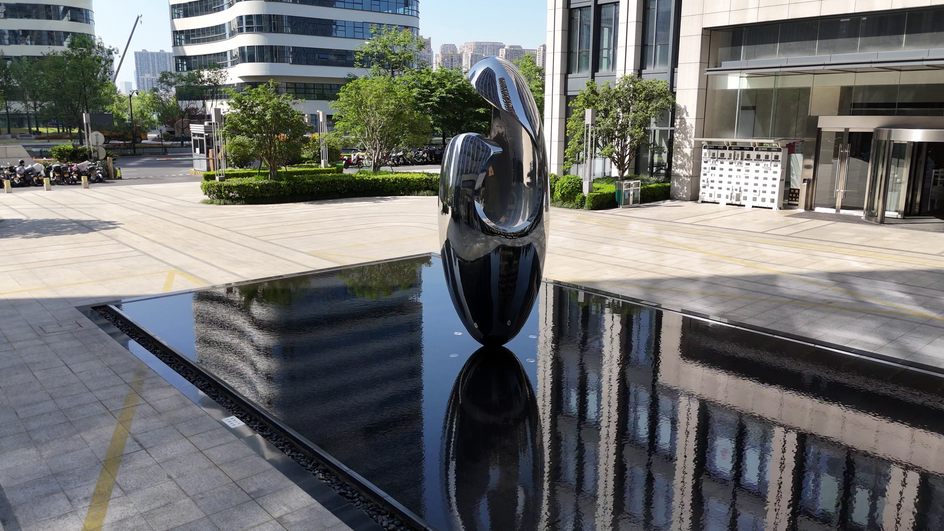
Rendering Results (Public Dataset)
We test our method on public datasets, including the RFF and Shiny datasets.
Method
We revisit ideas from geometry-guided ray interpolation techniques with modern neural fields, differentiable rendering, and end-to-end optimization. Rather than a global proxy geometry, we propose for each input view to define a local proxy geometry. As each local geometry only has to remain consistent across the small set of neighboring views used to produce a novel view, this makes it possible to represent complex curved reflectors in a `piecewise' way, helping to maintain sharp reflections.
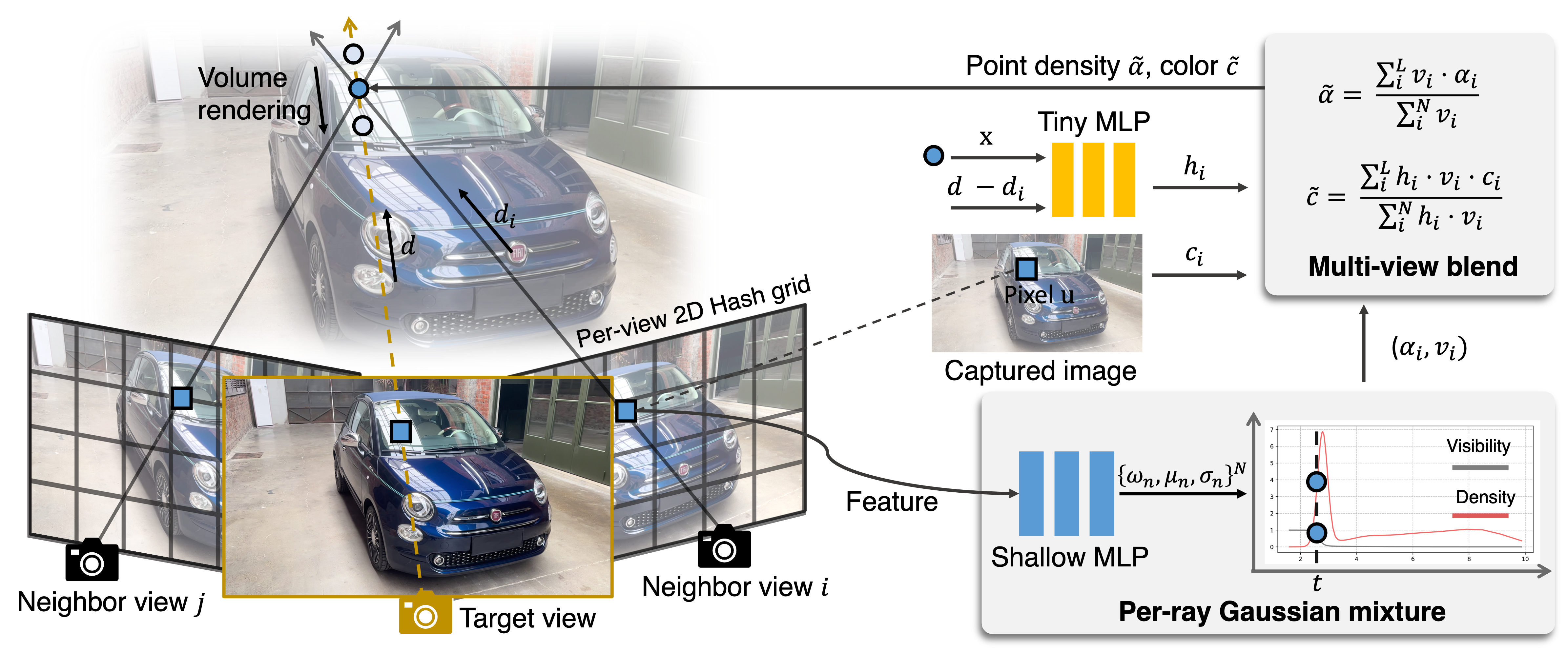
Comparisons
Comparisons with other state-of-the-art (SOTA) methods.
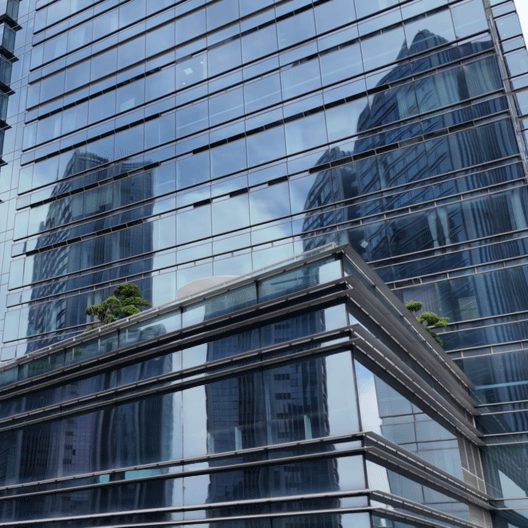
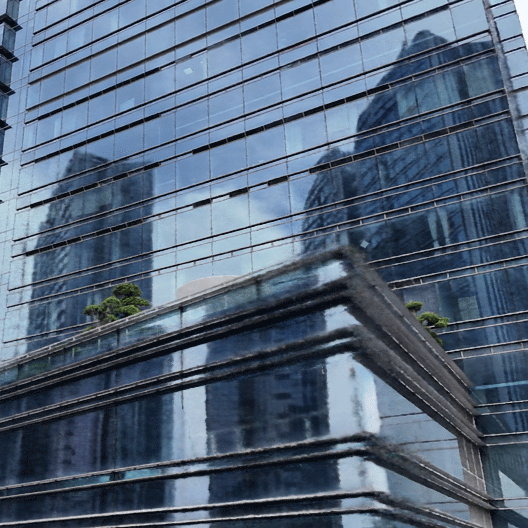
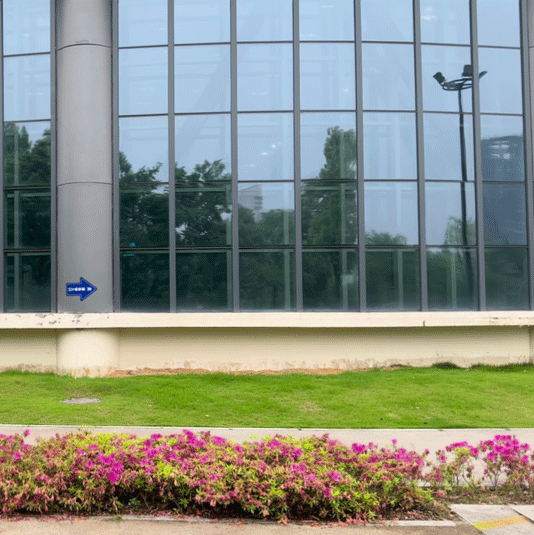
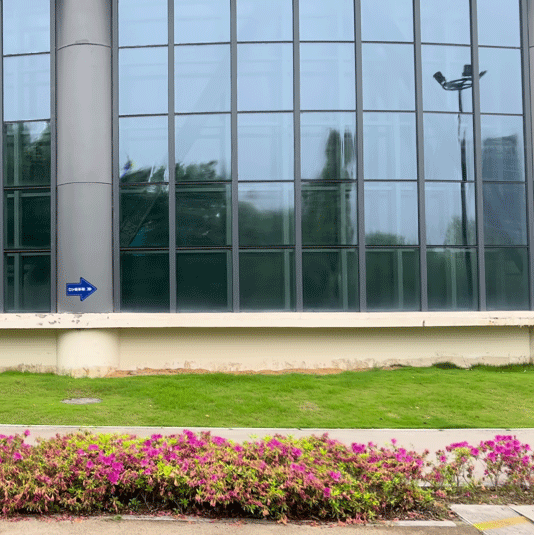
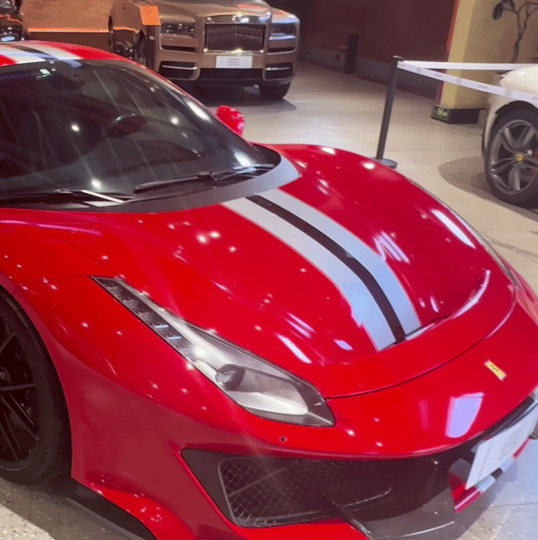
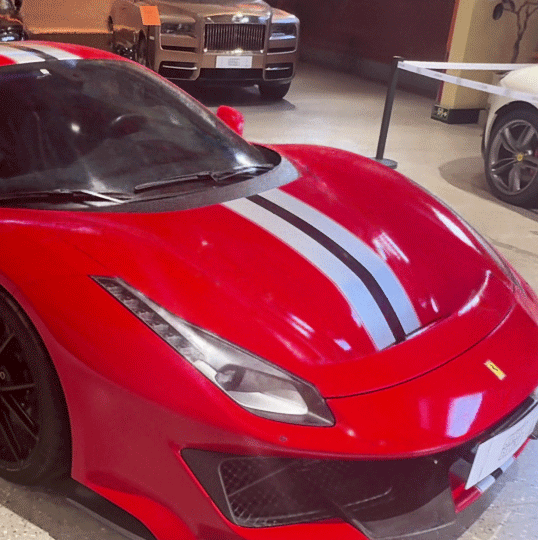
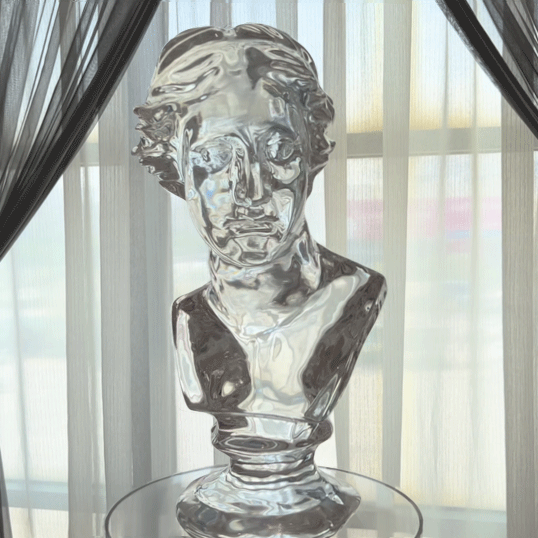
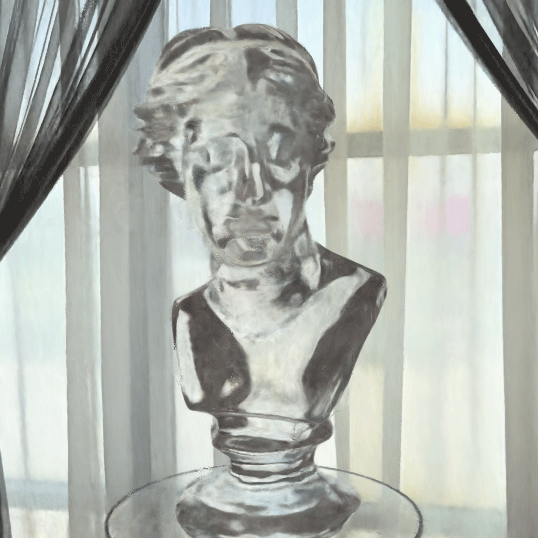
Full Video
Download (Coming Soon)
Download our paper, code, and dataset.
BibTex
@article{wu2024lgdm,
title={Local Gaussian Density Mixtures for Unstructured Lumigraph Rendering},
author={Wu, Xiuchao and Xu, Jiamin and Wang, Chi and Peng, Yifan and Huang, Qixing and Tompkin, James and Xu, Weiwei},
booktitle = {ACM SIGGRAPH Asia 2024 Conference Papers},
year = {2024},
}
Acknowledgements
Supported by Information Technology Center and State Key Lab of CAD&CG, Zhejiang University.